1.7. Radioactive lighthouse problem¶
Goal: Explore a classic problem from Gull (by way of Sivia’s book with some tweaks).
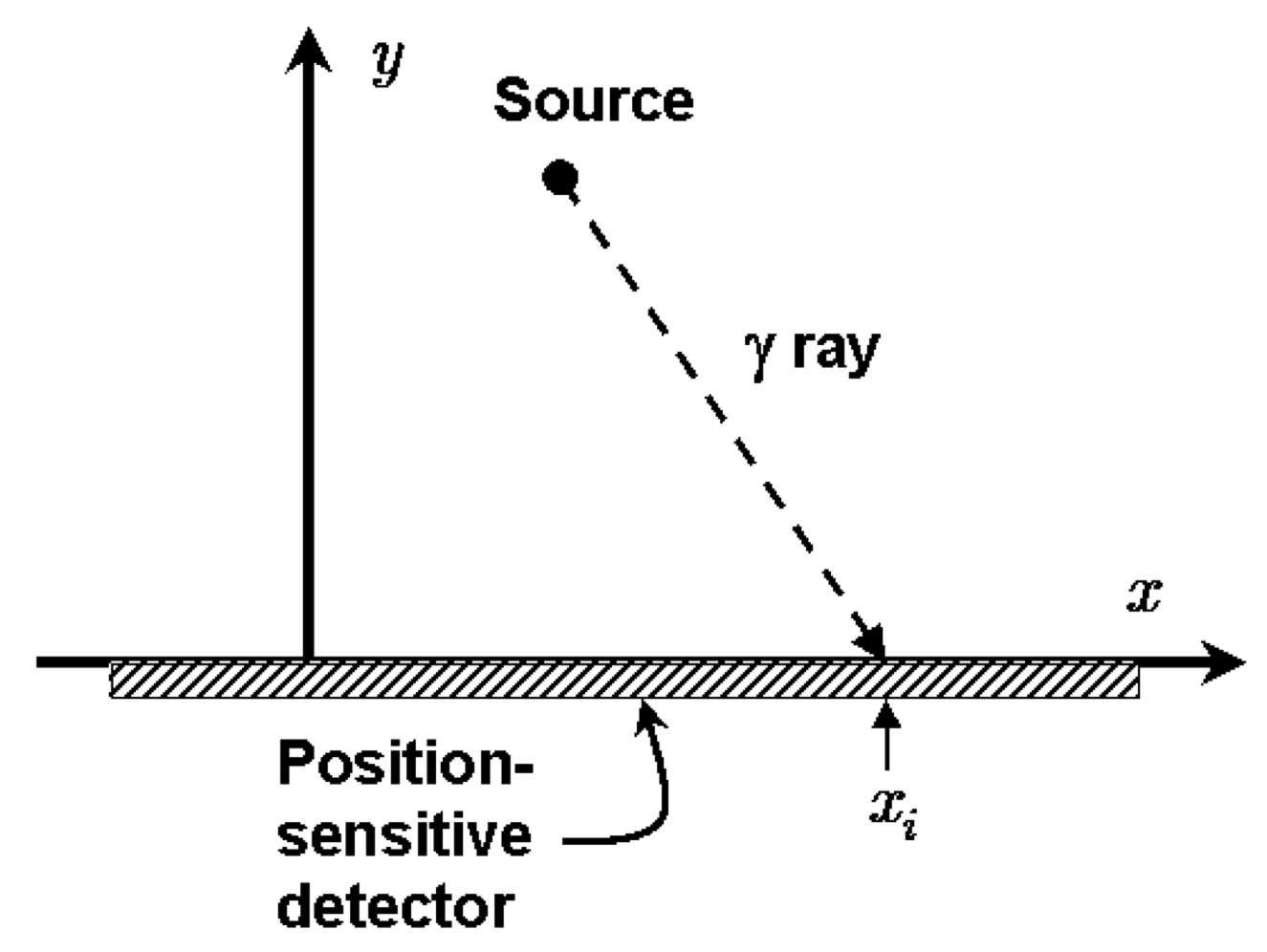
In the figure, a radioactive source that emits gamma rays randomly in time but uniformly in angle is placed at \((x_0, y_0)\). The gamma rays are detected on the \(x\)-axis and these positions are saved, \(x_k\), \(k=1,2,\cdots, N\). Given these observed positions, the problem is to estimate the location of the source.
Initially we’ll assume that we know that \(y_0 = 1\) (in whatever length units we are implicitly using), so our goal is to estimate \(x_0\). We introduce the angle \(\theta\) between the \(\gamma\) ray and the \(y\)-axis (with \(\theta = 0\) meaning the gamma ray is detected at \(x_0\)).
Refs:
D.S. Sivia, Data Analysis, A Bayesian Tutorial
S.F. Gull, Bayesian Inductive Inference and Maximum Entropy
\(% Some LaTeX definitions we'll use. \newcommand{\pr}{p} \newcommand{\xmax}{x_{0,\textrm{max}}} \newcommand{\xmin}{x_{0,\textrm{min}}} \newcommand{\Nmax}{N_{\textrm{max}}}\)
Answer the questions in italics. Check with your neighbors and ask for help if you get stuck or are unsure.
Claim: in the \(\pr(\cdot|\cdot)\) notation, our goal is to find the posterior pdf \(\pr(x_0 | \{x_k\}, y_0)\). How would you translate this posterior to words?
By Bayes’ theorem, how is this posterior related to \(\pr(\{x_k\} | x_0, y_0)\), \(\pr(x_0 | y_0)\), and \(\pr(\{x_k\}|y_0)\)?
Claim: because the denominator pdf in 2. is independent of \(x_0\), it is just a normalization factor for \(\pr(x_0 | \{x_k\}, y_0)\), so we don’t need to calculate it explicitly. Do you understand this? What good is an unnormalized posterior \(\pr(x_0 | \{x_k\}, y_0)\)?
Let’s take for the prior pdf \(\pr(x_0 | y_0)\) that
\[\pr(x_0 | y_0) = \pr(x_0) = \frac{1}{|\xmax - \xmin|} \quad\mbox{for}\ \xmin < x_0 < \xmax \]and zero elsewhere. What are we assuming? Why is this more plausible than letting \(x_0\) be anything? Why do we assume a constant pdf? Is this pdf normalized?
If we assume that the \(x_k\)s are independent, then how is \(\pr(\{x_k\}|x_0, y_0)\) simplified? Is this a justifiable assumption?
Show that for a particular \(k\),
\[ \pr(x_k|x_0, y_0) = \frac{y_0}{\pi} \frac{1}{y_0^2 + (x_k - x_0)^2} \;, \]given that the angular distribution from \(\theta_k\) is uniform from \(-\pi/2\) to \(\pi/2\), so \(\pr(\theta_k|x_0,y_0) = 1/\pi\), and also that
\[ \pr(\theta_k|x_0, y_0)\, d\theta_k = \pr(x_k | x_0, y_0)\, dx_k \;.\]Why is the latter true?
Ok, now we’re ready to see what the estimates for \(x_0\) look like. Use the following code to generate a set of random \(x\) points for a Cauchy distribution. Look up the Stats documentation for a Cauchy distribution (google “scipy stats cauchy”) to verify it is the same function derived above (note the use of
loc
andscale
). Run it a few times to see the fluctuations in the distribution.
What can you say about the tails of this distribution compared to your experience with Gaussian distributions?
%matplotlib inline
import numpy as np
import scipy.stats as stats
from scipy.stats import cauchy, uniform
import matplotlib.pyplot as plt
import seaborn as sns; sns.set() # for plot formatting
#sns.set_context("talk")
# True location of the radioactive source
x0_true = 1.
y0_true = 1.
# Generate num_pts random numbers distributed according to dist and plot
num_pts = 512
x_pts = np.arange(num_pts)
# Distribution knowing where the source is: scipy.stats.cauchy(loc, scale)
dist = cauchy(x0_true, y0_true)
dist_pts = dist.rvs(num_pts)
# Make some plots!
fig = plt.figure(figsize=(15,5))
# First plot all the points, letting it autoscale the counts
ax_1 = fig.add_subplot(1,3,1)
ax_1.scatter(x_pts, dist_pts)
# Repeat but zoom in to near the origin
ax_2 = fig.add_subplot(1,3,2)
ax_2.scatter(x_pts, dist_pts)
ax_2.set_ylim(-10.,10.)
# Finally make a zoomed-in histogram
ax_3 = fig.add_subplot(1,3,3)
out = ax_3.hist(dist_pts, bins=np.arange(-10., 10., 0.2))
# Print out the numerical limits (max and min)
print('maximum = ', np.amax(dist_pts))
print('minimum = ', np.amin(dist_pts))
fig.tight_layout()
8. Now you’ll repeat the same graphs, but this time generate the distribution of points starting with a uniform distribution in angle between \(-\pi/2\) and \(\pi/2\). In particular, at the ###
, write the formula for dist_pts
in terms of theta_dist
and x0_true
, y0_true
.
# True location of the radioactive source
x0_true = 1.
y0_true = 1.
# Generate num_pts random numbers distributed according to dist and plot
num_pts = 512
x_pts = np.arange(num_pts)
# Uniform distribution in theta: uniform(a,b) in [a, a+b]
theta_dist = uniform(-np.pi/2., np.pi)
#dist_pts_alt = ### Fill in formula here for x_k points
# Make some plots!
fig = plt.figure(figsize=(15,5))
# First plot all the points, letting it autoscale the counts
ax_1 = fig.add_subplot(1,3,1)
ax_1.scatter(x_pts, dist_pts_alt)
# Repeat but zoom in to near the origin
ax_2 = fig.add_subplot(1,3,2)
ax_2.scatter(x_pts, dist_pts_alt)
ax_2.set_ylim(-10.,10.)
# Finally make a zoomed-in histogram
ax_3 = fig.add_subplot(1,3,3)
out = ax_3.hist(dist_pts_alt, bins=np.arange(-10., 10., 0.2))
# Print out the numerical limits (max and min)
print('maximum = ', np.amax(dist_pts_alt))
print('minimum = ', np.amin(dist_pts_alt))
fig.tight_layout()
Before moving on, let’s do some more plotting of histograms with different numbers of samples to build some intuition. We define a plotting function first so it is easy to plot several histograms all at once. Run it several times to see the nature of the fluctuations.
def dist_hist_plot(ax, name, x_dist, dist, num_samples, bin_width,
x_label=None):
"""
Plot a pdf and histogram of samples with specified list of points to
be plotted, which sets the range of the histogram, and width of bins.
Parameters:
-----------
ax (matplotlib axis): axis for the histogram
name (string): description of
x_dist (ndarray): points to be plotted
dist (scipy.stats distribution): pdf to make draws from
num_samples (int): number of draws to make from dist
bin_width (float): width of each bin to be plotted
x_label (string): label for the x-axis
"""
samples = dist.rvs(size=num_samples) # generate num_samples draws
bin_bounds = np.arange(x_dist[0], x_dist[-1], bin_width)
count, bins, ignored = ax.hist(samples, bins=bin_bounds, density=True,
color='blue', alpha=0.7)
ax.plot(x_dist, dist.pdf(x_dist), linewidth=2, color='r')
title_string = name + f' samples = {num_samples:d}'
ax.set_title(title_string)
ax.set_xlim(x_dist[0], x_dist[-1])
if x_label:
ax.set_xlabel(x_label)
x_max = 10.
x_dist = np.linspace(-x_max, x_max, 500)
name = rf'cauchy $x_0=${x0_true:1.1f}, $y_0=${y0_true:1.1f}'
fig = plt.figure(figsize=(15,5))
bin_width = 0.5
x_label = r'$x_k$'
num_samples = 100
cauchy_dist = stats.cauchy(x0_true, y0_true)
ax_1 = fig.add_subplot(1, 3, 1)
dist_hist_plot(ax_1, name, x_dist, cauchy_dist, num_samples,
bin_width, x_label)
num_samples = 1000
cauchy_dist = stats.cauchy(x0_true, y0_true)
ax_2 = fig.add_subplot(1, 3, 2)
dist_hist_plot(ax_2, name, x_dist, cauchy_dist, num_samples,
bin_width, x_label)
num_samples = 10000
cauchy_dist = stats.cauchy(x0_true, y0_true)
ax_3 = fig.add_subplot(1, 3, 3)
dist_hist_plot(ax_3, name, x_dist, cauchy_dist, num_samples,
bin_width, x_label)
fig.tight_layout()
Define and plot the posterior for \(x_0\)¶
9. In this section the posterior for \(x_0\) is calculated and plotted for different numbers of data. The prior is taken to be a uniform PDF from \(-4\) to \(4\) (we really don’t believe it is bigger than that but otherwise we don’t know what it is). For each \(\Nmax\), besides plotting the posterior for \(x_0\), we calculate the mean of the posterior (denoted \(\langle x_0\rangle\)) and the mean of the set of \(\Nmax\) points (denoted \(\overline x_0\)).
def log_prior(x0, y0_true, x_min=-4., x_max=+4.):
"""
Log uniform prior from x_min to x_max. Not normalized!
"""
if (x0 > x_min) and (x0 < x_max):
return 0.
else:
return -np.inf # log(0) = -inf
def log_likelihood(x0, y0_true, dist_pts, N_max):
"""
Log likelihood for the first N_max points of the dist_pts array,
assuming independent. Not normalized!
"""
L_pts = -np.log(y0_true**2 + (dist_pts[0:N_max] - x0)**2)
return sum(L_pts)
def posterior_calc(x0_pts, y0_true, dist_pts, N_max, x0_min=-4., x0_max=+4.):
"""
Calculate the posterior for a set of x0_pts given y0 (y0_true) and a
list of N_max x_k observations (dist_pts).
"""
log_L_pts = [log_likelihood(x0, y0_true, dist_pts, N_max) \
for x0 in x0_pts]
log_L_pts -= np.amax(log_L_pts) # subtract maximum of log likelihood
log_prior_pts = [log_prior(x0, y0_true, x0_min, x0_max) \
for x0 in x0_pts]
posterior_pts = np.exp(log_prior_pts + log_L_pts)
return posterior_pts
def lighthouse_stats(dist_pts, N_max, x0_pts, posterior_pts):
"""
Given an array of N_max observed detection points (dist_pts) and a
posterior pdf (posterior_pts) for an array of x0 points (x0_pts), return
the mean of dist_pts and the mode and mean of the posterior.
"""
mean_dist = np.mean(dist_pts[0:N_max])
max_posterior = x0_pts[np.argmax(posterior_pts)]
mean_posterior = np.sum(x0_pts * posterior_pts) / np.sum(posterior_pts)
return mean_dist, max_posterior, mean_posterior
Some questions about the implementation through these functions:
If you wanted
log_prior
to return the normalized log prior, how would you modify the function?Why is the log likelihood adjusted in
posterior_calc
?Why is it not necessary to normalize the posterior? Would it not be easier just to normalize it?
# True location of the radioactive source
x0_true = 1.
y0_true = 1.
# Distribution knowing where the source is: scipy.stats.cauchy(loc, scale)
num_pts = 512 # number of observations
dist = cauchy(x0_true, y0_true) # sampling a Cauchy distribution directly
dist_pts = dist.rvs(num_pts) # generate {x_k} for k = 1 to num_pts
# Choose the set of N_max to be plotted (multiple of 3, up to num_pts)
N_max_values = [1, 2, 4, 8, 16, 64, 128, 256, 512]
x0_min = -4. # lower bound for prior
x0_max = +4. # upper bound for prior
x0_pts = np.arange(-4.5, 4.5, 0.01)
fig = plt.figure(figsize=(14, 2.5*len(N_max_values)/3))
# Step through counter (k) for each N_max entry in N_max_values
for k, N_max in enumerate(N_max_values):
posterior_pts = posterior_calc(x0_pts, y0_true, dist_pts, N_max)
mean_dist, max_posterior, mean_posterior = lighthouse_stats(dist_pts,
N_max, x0_pts, posterior_pts)
# now make the plots: 3 to a row
ax = fig.add_subplot(int(len(N_max_values)/3), 3, k+1)
ax.set_xlabel(r'source position $x_0$')
#ax.set_yticks([]) # turn off the plotting of ticks on the y-axis
ax.plot(x0_pts, posterior_pts, color='blue')
ax.set_title(rf'$N_{{\rm max}} = {N_max:d}$')
ax.axvline(mean_dist, 0., 1.1, color='black', linestyle="--", lw=2)
ax.axvline(mean_posterior, 0., 1.1, color='red', linestyle="--", lw=2)
ax.set_xlim(np.min(x0_pts), np.max(x0_pts))
stats_title = rf'$\overline{{x}}_0 = {mean_dist:.2f}$' + '\n' \
rf'$\langle x_0 \rangle = {mean_posterior:.2f}$'
ax.annotate(stats_title,
xy=(0.05,0.85), xycoords='axes fraction',
horizontalalignment='left',verticalalignment='top')
figure_title = rf'Radioactive lighthouse problem (true $x_0 = {x0_true:.1f}$)'
fig.suptitle(figure_title, y=1.02, fontsize=14)
fig.tight_layout()
Run the cell above several times for each \(\Nmax\) and record the results in the table:
%%html
<style>
table { width:80% !important; }
table td, table th, table tr {border: 2px solid black !important;
text-align:center !important;
font-size: 20px;}
</style>
\(\Nmax\) |
1: \(\langle x_0\rangle\) |
1: \(\overline x_0\) |
2: \(\langle x_0\rangle\) |
2: \(\overline x_0\) |
3: \(\langle x_0\rangle\) |
3: \(\overline x_0\) |
---|---|---|---|---|---|---|
1 |
|
|
|
|
|
|
2 |
|
|
|
|
|
|
4 |
|
|
|
|
|
|
16 |
|
|
|
|
|
|
64 |
|
|
|
|
|
|
256 |
|
|
|
|
|
|
10. What are your observations about the posterior for \(x_0\) as a function of \(\Nmax\)? Which mean (from the set of \(\Nmax\) points or from the posterior) is the better estimate?
11. Why does the Central Limit Theorem appear to fail? (The mean from the set of \(\Nmax\) samples does not tend to the true \(x_0\).)
Additional tasks:
Convert to sample the distribution using MCMC.
Generalize to find the joint posterior of \(x_0\) and \(y_0\).
(suggest something!)