2.7. Lecture 6¶
Follow-up to priors¶
Return to the discussion of priors for fitting a straight line.
Where does \(p(m) \propto 1/(1+m^2)^{3/2}\) come from?
We can consider the line to be given by \(y = m x + b\) or \(x = m' y + b'\), with \(m' = 1/m\) and \(b' = -b/m\). These give the same results.
But because the labeling is arbitrary, we expect (and will require) that the prior on \(m,b\) (i.e., \(p(m,b)\)) has the same functional form as those on \(m',b'\) (so \(p(m',b')\) with the same function \(p\)). Then for the probabilities, we must have
Evaluating the Jacobian gives \(1/m^3\), so we need to solve the functional equation:
A solution is
with \(c\) chosen to normalize the pdf. Check:
It works! Note that this prior is independent of \(b\).
How would you solve this without guessing the answer? Is there more than one solution?
[fill in answer]
Sivia example on “signal on top of background”¶
See [SS06] for further details. The figure shows the set up:
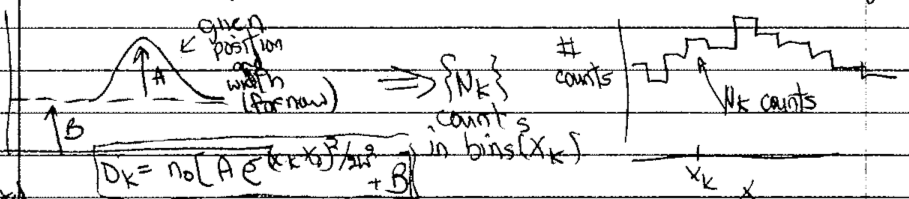
The level of the background is \(B\), the peak height of the signal above the background is \(A\) and there are \(\{N_k\}\) counts in the bins \(\{x_k\}\). The distribution is
Goal: Given \(\{N_k\}\), find \(A\) and \(B\).
So what is the posterior we want?
The actual counts we get will be integers, and we can expect a Poisson distribution:
or, with \(n\rightarrow N_k\), \(\mu \rightarrow D_k\),
for the \(k^{\text{th}}\) bin at \(x_k\).
What do we learn from the plots of the Poisson distribution?
which means that
Choose the constant for convenience; it is independent of \(A,B\).
Best point estimate: maximize \(L(A,B)\) to find \(A_0,B_0\)
Look at code for likelihood and prior
Uniform (flat) prior for \(0 \leq A \leq A_{\text{max}}\), \(0 < B \leq B_{\text{max}}\)
Not sensitive to \(A_{\text{max}}\), \(B_{\text{max}}\) if larger than support of likelihood
Table of results
Fig. # |
data bins |
\(\Delta x\) |
\((x_k)_{\text{max}}\) |
\(D_{\text{max}}\) |
---|---|---|---|---|
1 |
15 |
1 |
7 |
100 |
2 |
15 |
1 |
7 |
10 |
3 |
31 |
1 |
15 |
100 |
4 |
7 |
1 |
3 |
100 |
Comments on figures:
Figure 1: 15 bins and \(D_{\text{max}} = 100\)
Contours are at 20% intervals showing height
Read off best estimates and compare to true
does find signal is about half background
Marginalization of \(B\)
What if we don’t care about \(B\)? (“nuisance parameter”)
\[ p(A | \{N_k\}, I) \int_0^\infty p(A,B|\{N_k\},I)\, dB \]compare to \(p(A | \{N_k\}, B_{\text{true}}, I)\) \(\Longrightarrow\) plotted on graph
Also can marginalize over \(A\)
\[ p(B | \{N_k\}, I) \int_0^\infty p(A,B|\{N_k\},I)\, dA \]Note how these are done in code:
B_marginalized
andB_true_fixed
, and note the normalization at the end.
Set extra plots to true
different representations of the same info and contours in the first three. The last one is an attempt at 68%, 95%, 99.7% (but looks wrong).
note the difference between contours showing the pdf height and showing the integrated volume.
Look at the other figures and draw conclusions.
How should you design your experiments?
E.g., how should you bin data, how many counts are needed, what \((x_k)_{\text{max}}\), and so on.