2.4. Lecture 5¶
Follow-up to Gaussian approximation
We motivated Gaussian approximations from a Taylor expansion to quadratic order of the logarithm of a pdf. What could go wrong if we directly expanded the pdf?
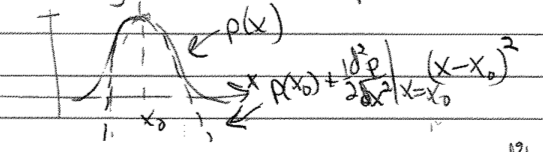
A pdf must be normalizable and positive definite, so this approximation violates these conditions!
In contrast,
where we note that the second derivative is less than zero so the exponent is negative definite.
Note that this approximation is not only positive definite and normalizable, it is a higher-order approximation to \(p(x)\) because it includes all orders in \((x-x_0)^2\).
Compare Gaussian noise sampling to lighthouse calculation¶
Jump to the Bayesian approach in Parameter estimation example: Gaussian noise and averages and then come back to contrast with the frequentist approach.
Compare the goals between the Gaussian noise sampling (left) and the radioactive lighthouse problem (right): in both cases the goal is to sample a posterior \(p(\thetavec|D,I)\)
\[ p(\mu,\sigma | D, I) \leftrightarrow p(x_0,y_0 | D, I) \]where \(D\) on the left are the \(x\) points and \(D\) on the right are the \(\{x_k\}\) where flashes hit.
What do we need? From Bayes’ theorem, we need
\[\begin{split}\begin{align} \text{likelihood:}& \quad p(D|\mu,\sigma,I) \leftrightarrow p(D|x_0,y_0,I) \\ \text{prior:}& \quad p(\mu,\sigma|I) \leftrightarrow p(x_0,y_0|I) \end{align}\end{split}\]You are generalizing the functions for log pdfs and the plotting of posteriors that are in notebook Radioactive lighthouse problem Key.
Note in Parameter estimation example: Gaussian noise and averages the functions for log-prior and log-likelihood.
Here \(\thetavec = [\mu,\sigma]\) is a vector of parameters; cf. \(\thetavec = [x_0,y_0]\).
Step through the set up for
emcee
.It is best to create an environment that will include
emcee
andcorner
.
Hint
Nothing in the
emcee
sampling part needs to change!More later on what is happening, but basically we are doing 50 random walks in parallel to explore the posterior. Where the walkers end up will define our samples of \(\mu,\sigma\) \(\Longrightarrow\) the histogram is an approximation to the (unnormalized) joint posterior.
Plotting is also the same, once you change labels and
mu_true
,sigma_true
tox0_true
,y0_true
. (And skip themaxlike
part.)
Analysis:
Maximum likelihood here is the frequentist estimate \(\longrightarrow\) this is an optimization problem.
Question
Are \(\mu\) and \(\sigma\) correlated or uncorrelated?
Answer
They are uncorrelated because the contour ellipses in the joint posterior have their major and minor axes parallel to the \(\mu\) and \(\sigma\) axes. Note that the fact that they look like circles is just an accident of the ranges chosen for the axes; if you changed the \(\sigma\) axis range by a factor of two, the circle would become flattened.
Read off marginalized estimates for \(\mu\) and \(\sigma\).
Central limit theorem (CLT)¶
Let’s start with a general characterization of the central limit theorem (CLT):
General form of the CLT
The sum of \(n\) random variables that are drawn from any pdf(s) of finite variance \(\sigma^2\) tends as \(n\rightarrow\infty\) to be Gaussian distributed about the expectation value of the sum, with variance \(n\sigma^2\). (So we scale the sum by \(1/\sqrt{n}\); see below.)
Consequences:
The mean of a large number of values becomes normally distributed regardless of the probability distribution from which the values are drawn. (Why does this fail for the lighthouse problem?)
Functions such as the Binomial and Poisson distribution all tend to Gaussian distributions with a large number of draws.
so mean \(\mu = \lambda\) and variance \(\sigma^2 = \lambda\) (variance, not the standard deviation).
Class questions
i) How would you verify this in a Jupyter notebook?
ii) How would you prove it analytically?
Answer
[add an answer]
Proof in special case¶
Start with independent random variables \(x_1,\cdots,x_n\) drawn from a distribution with mean \(\langle x \rangle = 0\) and variance \(\langle x^2\rangle = \sigma^2\), where
(generalize later to nonzero mean). Now let
(we scale by \(1/\sqrt{n}\) so that \(X\) is finite in the \(n\rightarrow\infty\) limit).
What is the distribution of \(X\)? \(\Longrightarrow\) call it \(p(X|I)\), where \(I\) is the information about the probability distribution for \(x_j\).
Plan: Use the sum and product rules and their consequences to relate \(p(X)\) to what we know of \(p(x_j)\). (Note: we’ll suppress \(I\) to keep the formulas from getting too cluttered.)
Class: state the rule used to justify each step
marginalization
product rule
independence
We might proceed by using a direct, normalized expression for \(p(X|x_1,\cdots,x_n)\):
Question
What is \(p(X|x_1,\cdots,x_n)\)?
Answer
\(p(X|x_1,\cdots,x_n) = \delta\Bigl(X - \frac{1}{\sqrt{n}}(x_1 + \cdots + x_n)\Bigr)\)
Instead we will use a Fourier representation:
Substituting into \(p(X)\) and gathering together all pieces with \(x_j\) dependence while exchanging the order of integrations:
Observe that the terms in []s have factorized into a product of independent integrals and they are all the same (just different labels for the integration variables).
Now we Taylor expand \(e^{i\omega x_j/\sqrt{n}}\), arguing that the Fourier integral is dominated by small \(x\) as \(n\rightarrow\infty\). (When does this fail?)
Then, using that \(p(x)\) is normalized (i.e., \(\int_{-\infty}^{\infty} dx\, p(x) = 1\)),
Now we can substitute into the posterior for \(X\) and take the large \(n\) limit:
Here we’ve used:
\(\lim_{n\rightarrow\infty}\left(1+\frac{a}{n}\right)^n = e^a\) with \(a = -\omega^2\sigma^2/2\).
\(\int_{-\infty}^{\infty} dz\, e^{-Az^2/2}e^{iBz} = \sqrt{2\pi/A} e^{-B^2/2A}\) with \(z = \omega\), \(A = \sigma^2\), and \(B = X\).
To generalize to \(\langle x \rangle \neq 0\) (non-zero mean), consider \(X = \bigl[(x_1 + \cdots x_n) - n\mu\bigr]/\sqrt{n}\) and change to \(y_j = x_j - \mu\). Now \(X\) is a sum of \(y_j\)’s and the proof goes through the same.
So the distribution of means of samples of size \(n\) from any distribution with finite variance becomes for large \(n\) a Gaussian with width equal to the standard deviation of the distribution divided by \(\sqrt{n}\).
Likelihoods (or posteriors) with two variables with quadratic approximation¶
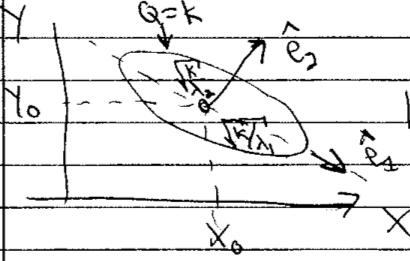
Find \(X_0\), \(Y_0\) (best estimate) by differentiating
To check reliability, Taylor expand around \(L(X_0,Y_0)\):
It makes sense to do this in (symmetric) matrix notation:
So in quadratic approximation, the contour \(Q=k\) for some \(k\) is an ellipse centered at \(X_0, Y_0\). The orientation and eccentricity are determined by \(A\), \(B\), and \(C\).
The principal axes are found from the eigenvectors of the Hessian matrix \(\begin{pmatrix} A & C \\ C & B \end{pmatrix}\).
What is the ellipse is skewed?
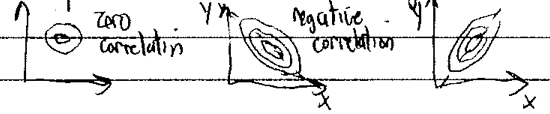
Look at correlation matrix